How Biologists are Using Machine Learning to Study Cells
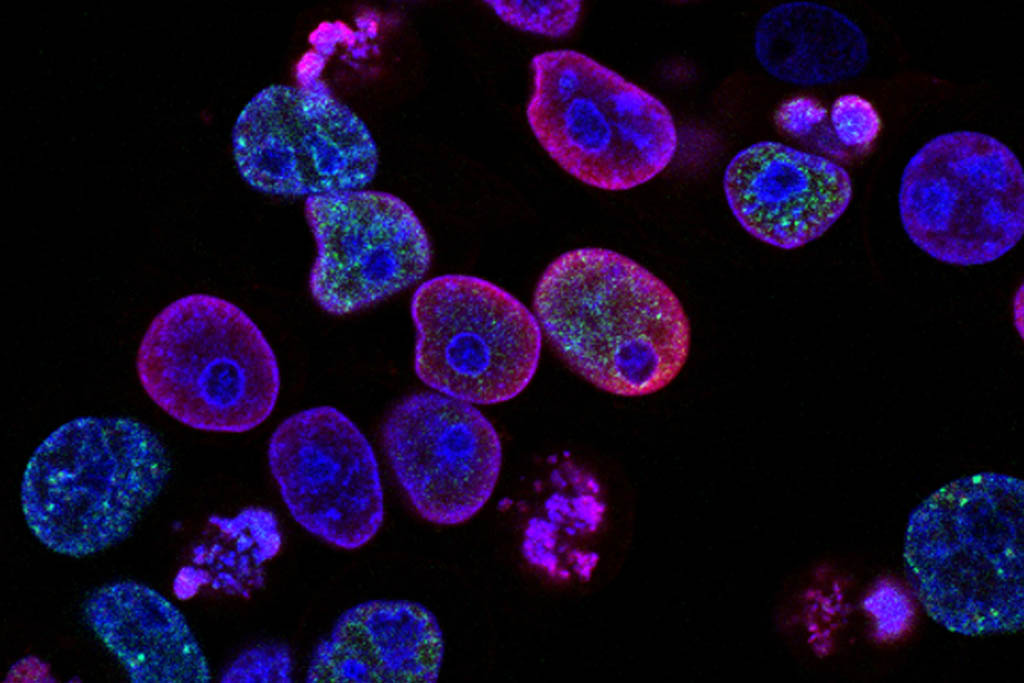
Anyone graduating with a biology degree will one day work closely with emerging machine learning (artificial intelligence) tools and algorithms.
Here are three life science questions biologists are exploring thanks to advancements in machine learning.
Can we model cell architecture and discover new cell parts?
Researchers at University of California San Diego School of Medicine, Stanford University and KTH Royal Institute of Technology in Stockholm, Sweden asked a fairly basic question: could we create a better map of human cell architecture?
Studying a human kidney cell line, the researchers found 69 subcellular systems — half of which had never been seen before. In one example, the researchers spotted complex of proteins that is likely involved in splicing and helps determine which genes are activated at which times.
Existing microscopes and biochemistry techniques produce important data. The computing power of machine learning can be trained to turn that data into a more complete model of the cell. Scientists hope to repeat the process with all human cells — healthy and diseased — and then compare. Such detail should reveal patterns key to understanding the molecular basis of many diseases.
Can we model cell behavior with more accuracy?
The key to understanding human cell behavior is to label as many of the parts as possible, and then watch how the parts interact over time.
Traditional fluorescence microscopy offers scientists a limited range of colored labels, and requires expensive reagents that damage the very cell scientists are trying to study. One alternative is called transmitted white light (bright-field microscopy), a technique that avoids the stains, but settles for a lower contrast, meaning most cell structures remain too difficult to spot.
Scientists from the Allen Institute for Cell Science in Seattle, Washington applied computing power to get the best of the two techniques. Artificial intelligence (AI) refined the bright-field images by applying a ‘virtual staining’ — allowing a better look inside the cell without the damaging effects of Traditional fluorescence microscopy.”
Can we predict how our cells will respond to therapeutic changes?
The great hope of regenerative medicine is the idea that our understanding of human cells might one day be so complete that drugs and other therapeutics can be personalized at the cellular level.
For now, science recognizes that differences happen from cell to cell and person to person. Scientists have a hard time predicting how cells will respond in any given therapeutic scenario.
Scientists from the National Institute of Standards and Technology and the National Eye Institute at the National Institutes of Health turned to deep learning to better match lab-grown eye tissue to a range of patients suffering from macular degeneration.
While humans struggle with the detailed observations and mathematical calculations required to compare terabytes of microscopic data, the machine learning tools proved to be relatively fast and accurate.
Your Future in Biology
Students interested in the cellular research made possible by machine learning can explore John Carroll University’s Cell and Molecular Biology degree. This program helps its students develop the skills needed to engage with exciting research opportunities such as these.
John Carroll is a private Jesuit university located in University Heights, Ohio, near Cleveland.