The future of artificial and collaborative intelligence in nursing is now.
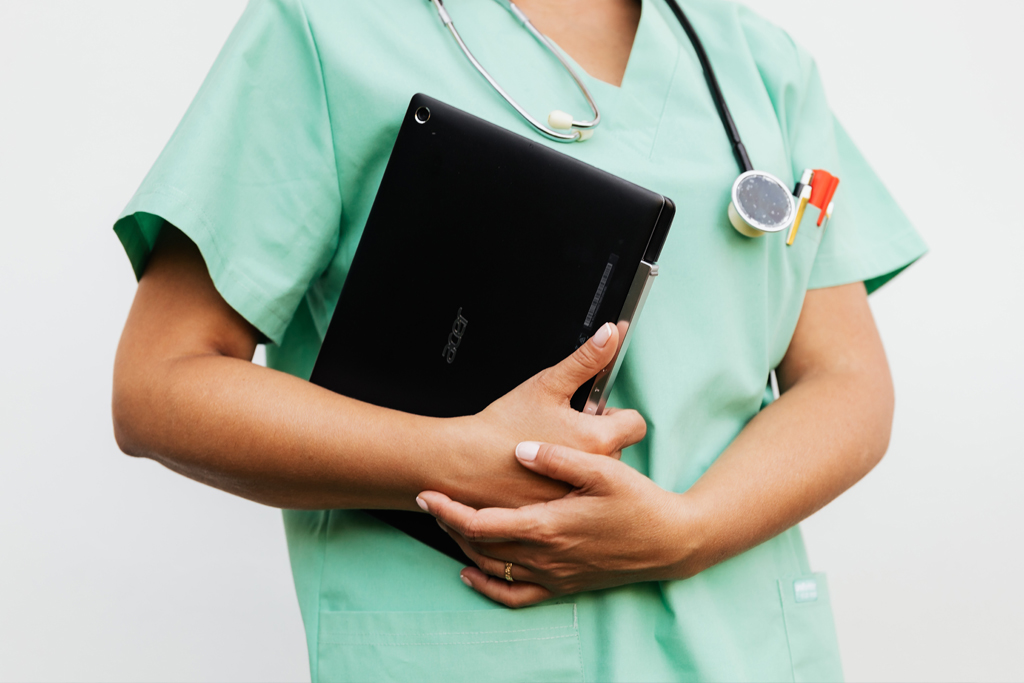
While direct care, hands-on clinical judgment, and patient empathy remain essential aspects of nursing, health care is changing.
Students enrolling in the John Carroll University Bachelors of Science in Nursing program in Cleveland, Ohio recognize that the future is now — collaboration between humans and various forms of artificial intelligence (AI) algorithms and tools will be a mainstay of nursing education and practice.
AI, Data Analysis and Machine Learning in Nursing
Application of data analysis and machine learning algorithms continues to expand in an effort to enhance diagnostics, treatment, and patient care. An early example is the Emergency Triage Systems software that can assist nurses in accurately triaging patients in emergency departments.
The Healthcare artificial intelligence market is expected to reach 280.77 billion by 2032, according to a research report published by Spherical Insights & Consulting.
A good example of how computational tools might one day analyze large volumes of medical information, including patient electronic health records and imaging data, to uncover valuable insights and support clinical decision-making is an experimental new platform from Glass Health. The tool foreshadows a day when nurses, doctors and others use a measure of automation to generate a clinical plan or differential diagnosis.
Practicing nurses today interact with a range of artificial intelligence (AI) algorithms and tools to manage everything from medication interactions, preventable falls, surgical complications, and dangerous infections.
This overview from My American Nurse gives nurses an overview of AI applications— machine learning, deep learning, and natural language processing (NLP)—and their implications for nursing research and practice, as well as their potential role in improving patient care and health outcomes.
Here’s a quick glossary of terms:
Machine Learning
Machine learning refers to the use of a computer program to autonomously learn from data to perform a certain task. The “learning” refers to software self-adjustment that fine-tunes an algorithm over time to increase accuracy. Like any data-dependent tool, a machine learning tool’s function and use are only as good as its data sources. Nurses with a boots-on-the-ground perspective understand patient care and the information that’s required to make informed clinical decisions. Nurse input improves the applicability and accuracy of machine learning tools.
Deep Learning
Deep learning, a subfield of machine learning, looks to use neural networks for advanced pattern recognition to predict, treat or prevent adverse health events such as infections, aneurysms or early onset of neurological disease. By integrating data from many sources, AI may someday be used to tailor treatments precisely to patients’ specific genes, lifestyles, and treatment preferences.
Natural Language Processing
Natural language processing allows for analysis of EHR text data, including nursing notes which are rich with clinical information. A wide variety of applications have been developed using nursing notes, including predicting emergency department patient disposition (Sterling and colleagues), uncovering patient financial barriers (Skaljic and colleagues), and predicting falls (Nakatani and colleagues).